CTRL: A CONDITIONAL TRANSFORMER LANGUAGE MODEL FOR CONTROLLABLE GENERATION
- 7 minsAuthors : Nitish Shirish Keskar, Bryan McCann, Lav R. Varshney, Caiming Xiong, Richard Socher by Salesforce Research
Paper : https://arxiv.org/pdf/1909.05858.pdf
Code : https://github.com/salesforce/ctrl
Summary
Control Code 를 이용하여 Language model 에 Condition을 주어 도메인, 스타일, 토픽 등과 같이 생성 되는 문장의 내용 및 스타일을 컨트롤 하여 생성 함
- Generation의 Control 가능성
- Conditional Transformer Language Model 사용
- Unsupervised learning (co-occurs)
Abstract
Large-scale language models show promising text generation capabilities, but users cannot easily control particular aspects of the generated text. We release CTRL, a 1.63 billion-parameter conditional transformer language model, trained to condition on control codes that govern style, content, and task-specific behavior. Control codes were derived from structure that naturally co-occurs with raw text, preserving the advantages of unsupervised learning while providing more explicit control over text generation. These codes also allow CTRL to predict which parts of the training data are most likely given a sequence. This provides a potential method for analyzing large amounts of data via model-based source attribution. We have released multiple full-sized, pretrained versions of CTRL at https://github.com/salesforce/ctrl.
1. Introduction
- Computer Vision 분야에서는 Generative adversarial networks가 image generation을 퍼포먼스를 상승 시켰으며, control 하는 연구도 진행 되었음
- Natural Language Processing 분야에서도 마찬가지로 conditional language model을 사용하여 text generation에 대한 연구 및 transfer learning 등 다양한 연구가 되었음.
- 이러한 연구로 인해 text generation을 제어 할 수 있는 방법에 대한 의문이 제기됨
- Conditional Transformer Language (CTRL) model 은 1.63 billion 의 파라미터를 가지며, specify domain, style, topics, dates, entities, relationships between entities, plot points, and task-related behavior의 control code에 따라 텍스트를 생성함
- Unsupervised learning 을 하기 위해서 원문에서 함께 등장하는 단어 (co-occur) 를 control code로 사용하여 학습함
- Ex ) 위키피디아, 아마존 리뷰 등은 주제 도메인과 관련된 단어, Reddit 은 r/ subdomain
- 이러한 방법을 통해 초기 프롬프트 (입력 context) 를 고정 시켜 두어도 control code에 따라서 예측 가능하게 생성 할 수 있음
- 모든 Control code는 training data의 subset으로 역추적이 가능하므로 모델 및 control code, training data 사이의 상관관계를 분석하는데 사용 될 수 있음
- Control code를 통해서 QA, machine translation과 같은 task도 수행할 수 있음
2. Language Modeling
- 기존의 Language Model :
-
텍스트 시퀀스 (\(x_1\), …, \(x_n\)) 으로 \(P(x)\) 를 학습
-
Next-word prediction : 주어진 dataset D = (\(x^1\), …, \(x^{\mid D\mid }\))를 이용, Negative Log-Likelihood 를 최소화 하여 parameters \(\theta\) 를 학습
- Language model은 \(p_\theta\)\((x_i\mid x_{<i})\)를 학습하기 때문에 length m을 갖는 새로운 \(\tilde{x}\) 를 생성 할 수 있음:
3. LANGUAGE MODELING WITH CTRL
- CTRL은 항상 control code c 를 바탕으로 \(p(x\mid c)\) 를 학습한다.
- Control code c 는 생성 과정에서 컨트롤 할 수 있도록 도와준다.
-
CTRL은 Control code가 raw text의 앞에 붙어서 \(p_θ (x_i\mid x<i,c)\) 가 학습된다.
ex) [Control Code] [Start] \(x_1\) \(x_2\) \(x_3\) … \(x_n\)
- 모델 구조는 Transformer Decoder를 쌓은 것과 유사함 (GPT2 와 유사)
- Softmax로 학습
3.1 DATA
- 총 140 GB 의 다양한 도메인 데이터로 학습함
- Wikipedia (En, De, Es, Fr)
- Project Gutenberg
- Submissions from 45 subreddits
- OpenWebText
- A large collection of news data
- Amazon Reviews
- Europarl and UN data from WMT (En-De, En-Es, En-Fr)
- Question-Answer Pair (no context documents) from ELI5
- MPQA shared task, which includes the Stanford Question Answering Dataset
- NewsQA
- Trivia QA
- SerachQA
- HotpotQA
-
Natural Questions
- Dataset에 대한 Control Code는 다음과 같음
3.2 EXPERIMENTAL SETTINGS
- FastBPE를 통한 BPE 학습 후 사용 (Large vocab : 250K tokens)
- 데이터가 2개 이상의 Unknown token을 갖으면 없앰 (180GB → 140GB 로 줄일 수 있었음)
- 각 텍스트는 도메인으로 부터 가져왔으며, 해당 도메인 컨트롤 코드를 텍스트의 앞 부분에 붙임
- 메모리와 최적화 제약으로 sequence length를 256과 512로 테스트 해본 결과 256이 512와 큰 차이가 나지 않아서 256을 사용
- 비슷한 접근 보다 vocab이 약 4배 더 크므로 실질적인 시퀀스 길이는 다른 접근 방식과 비슷함
- 기타 하이퍼 파라미터
- Dimension d = 1280
- Inner dimension f = 8192
- 48 layers and 16 heads per layer
- Dropout with probability 0.1 follows the residual connections in each layer
- Token embeddings were tied with the final output embedding layer
- Using Tensorflow
- Global batch size of 1024 distributed across 256 cores of a Cloud TPU v3 Pod for 800k iterations
- Training took approximately 2 weeks using Adagrad with a linear warmup from 0 to 0.05 over 25k steps
- The norm of gradients were clipped to 0.25
4. CONTROLLABLE GENERATION
4.1 SAMPLING
- Trained language model로 부터 sampling 할 때, 일반적으로 top-k sampling with temperature 를 함
- Top-k 후보에서 Distribution에 따라 sampling
-
T → 0 greedy distribution, T → ∞ uniform distribution
- top-k를 선택하는 방법으로는 Nucleus sampling이 존재함
- Threshold \(p_i\) 를 정하고 probability가 높은 토큰 부터 cumulative하여 \(p_i\)를 넘은 토큰까지를 top-k로 정함
- 이러한 방식을 사용하지 않고 penalized sampling을 사용
- Greedy에 가까운 sampling을 통해 기존 모델의 distribution을 신뢰 하면서도 반복 생성을 방지하는 방식
- 이전에 생성된 토큰 (g) 에 대해 score를 discounting 함
- Training 때는 사용하지 않음
- θ 를 1.2 쯤으로 하였을 때 정확한 토큰을 생성 하면서 반복 생성을 방지하는데 좋은 효과가 있음 (Heuristic)
4.2 CONTROL CODES
- Style by domain : training dataset의 domain으로 control code 사용 (Table 1, Table 2)
- More complex control codes : domain 외에 추가적으로 다른 control code를 사용. URL 및 subdomain 등. (Table 2, Table 3)
- Triggering specific tasks : 특정 task로 시작 함 (QA, Translation). MT-DNN / T5 방식과 동일 (Table 4)
- Zero-shot code-mixing : 다양한 control code를 한번에 사용하는 방식 (Table 2, table 5)
Toggle
Table 1
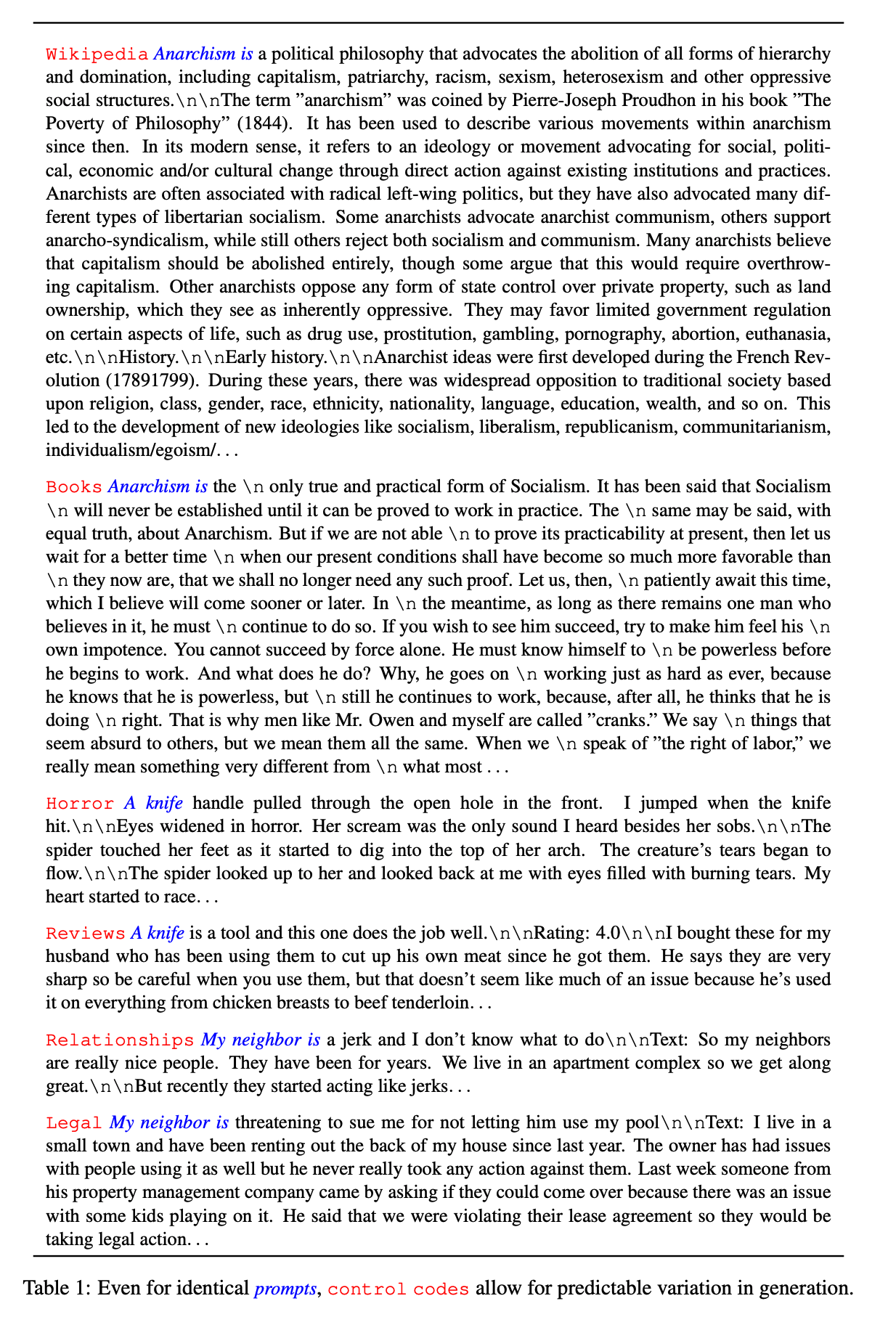
Table 2
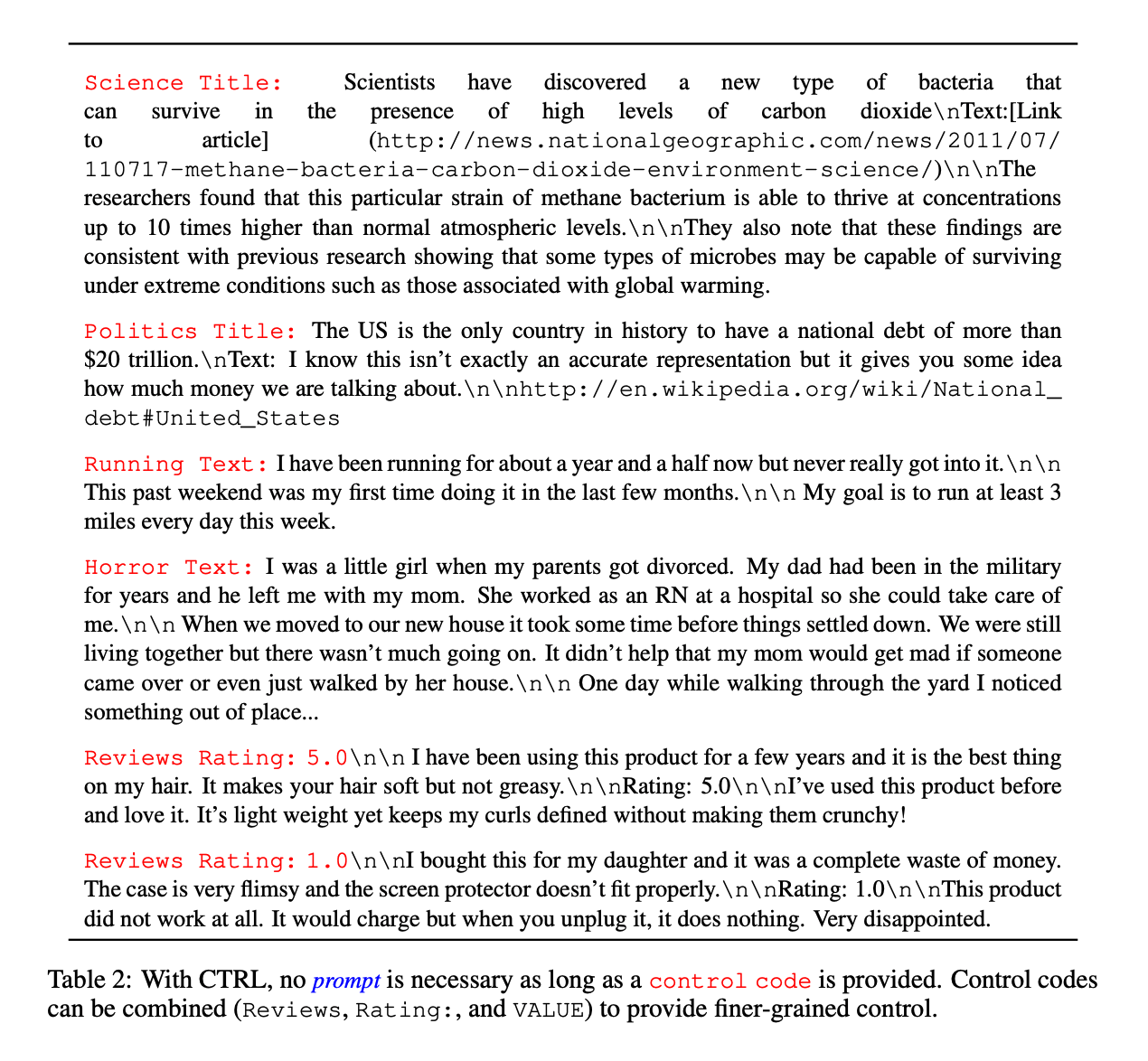
Table 3
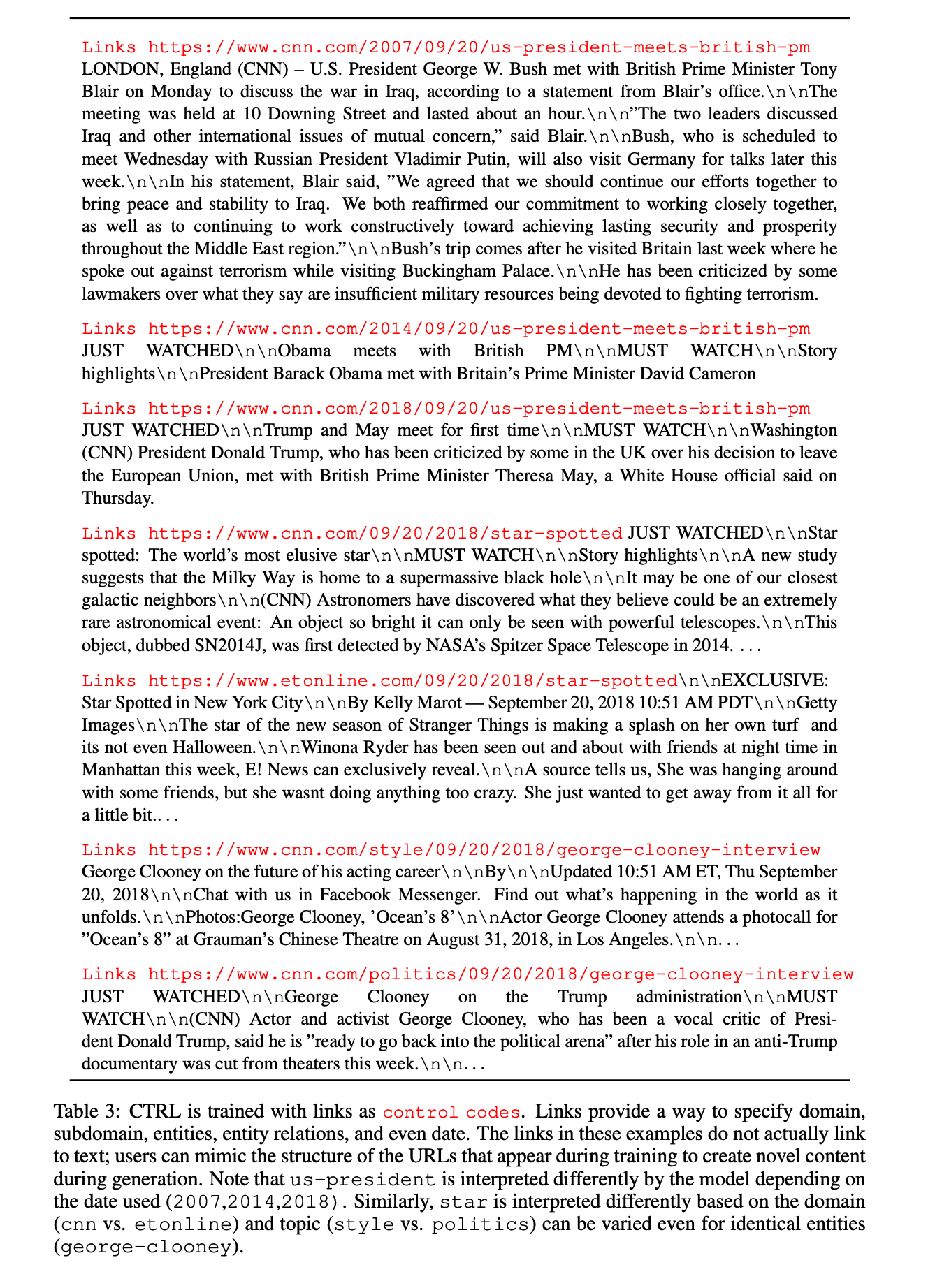
Table 4
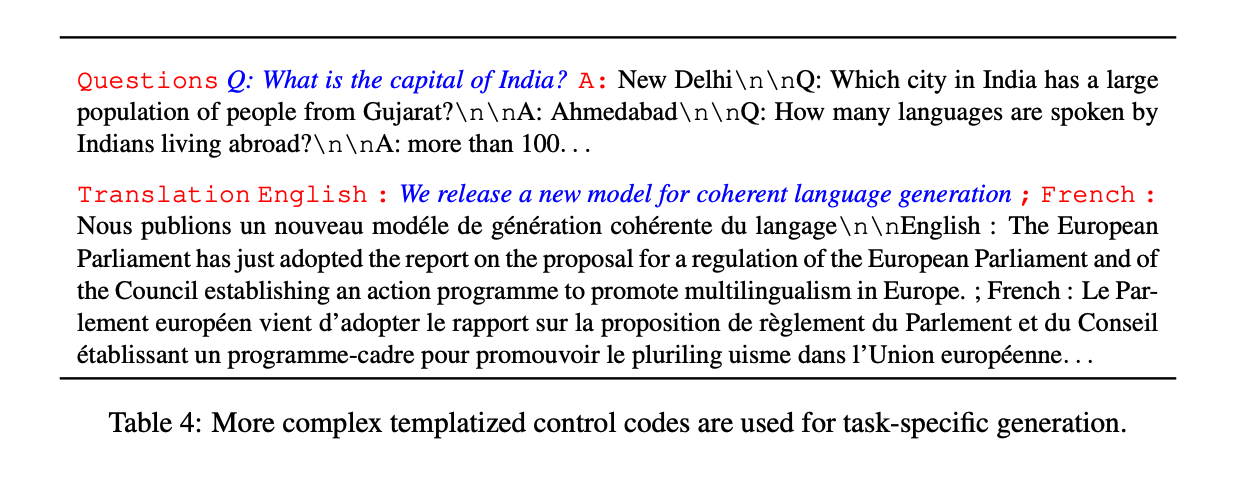
Table 5
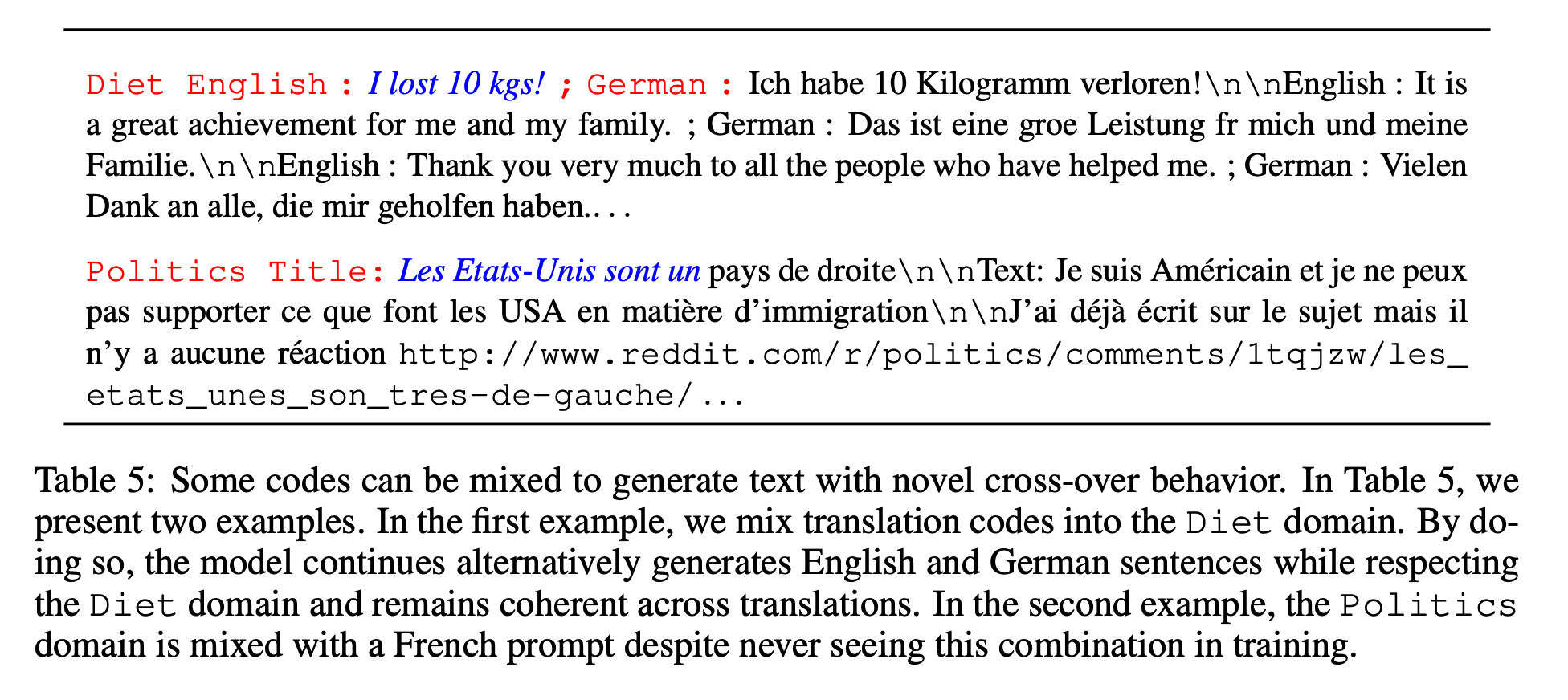
5. SOURCE ATTRIBUTION
- Domain control codes 는 training data를 mutually exclusive sets으로 나눌 수 있음
- 이는 어떤 training data의 subset이 language model에 의해 생성된 텍스트에 영향을 줬는지 알 수 있음
-
Language model은 $$p_θ(x c)$$ 를 학습하는 것 이다 (Recall) - Prior p(c)는 domain 에 대한 랭킹을 계산하는 straightforward 한 방법
- Training data의 empirical prior가 많은 데이터를 갖는 도메인에 많은 weight를 주는 것을 발견
- 따라서 uniform prior를 사용함
- Source attribution은 정확성(veracity)의 척도는 아니지만, 각 domain token이 생성된 텍스트에 얼마나 많은 영향을 주는 지에 대한 척도임
- 모델은 어떤 특정한 문화가 좋은지 나쁜지, 옳고 그른지, 진실인지 거짓 인지에 대한 개념을 가지고 있지 않음
- 단지 문화적인 연관성과 도메인 사이의 상관관계를 학습함
- CTRL은 특정 도메인이 주어진 진술과 유사한 언어를 포함 할 가능성이 더 높다는 모델 기반으로 evidence를 제공하지만 규범 적인 주장을 하는 데 사용해서는 안됨
- 많은 양의 텍스트에서 상관 관계를 분석 하기 위한 설명 도구임
6. RELATED WORK
7. FUTURE DIRECTIONS
- More control codes and finer-grained control.
- Extensions to other areas in NLP.
- Analyzing the relationships between language models and training data.
- Making the interface between humans and language models more explicit and intuitive.
8. CTRL-ALT-DEL: THE ETHICS OF LARGE LANGUAGE MODELS
생략
9. CONCLUSION
With 1.63 billion parameters, CTRL is the largest publicly released language model to date. It is trained with control codes so that text generation can be more easily controlled by human users. These codes allow users to explicitly specify domain, subdomain, entities, relationships between entities, dates, and task-specific behavior. We hope that the release of this model at https://github.com/salesforce/ctrl pushes towards more controllable, general models for natural language processing, and we encourage future discussion about artificial generation with our team by emailing ctrl- monitoring@salesforce.com.